Time Series Forecasting
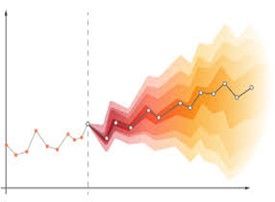
Time series forecasting is a methodology for estimating unknown future values of a variable based on its previous observations, with time being a significant factor. In contrast with standard predictive modeling and its related models, traditional ones concentrate on how the data is arranged over time in a sequence. In this regard, they are beneficial in giving an insight into the likely direction of some trends or sequences of events. The focus is to identify patterns like upward or downward trends over time, repeating cycles over time (seasonality), and correlations (the effects of past values on the current and future time intervals). Many sectors require forecasting. Time series models are applied to predict stock prices, interest rates, and foreign exchange rates in the financial industry. In the case of economics, these models are used to make predictions about factors such as GDP, unemployment, or inflation. Within the supply chain management domain, forecasts support resource planning, scheduling of production, and inventory control to ensure that demand is satisfied. Weather forecasting also utilizes time series forecasting methods; estimations of temperature, precipitation, or even storms seen at a particular geographical region are based on time series of the weather data for that region. Optimum forecasting can enable business organizations and governments to make sound decisions, minimize risks, and seize foresighted opportunities. Because of these factors, time series forecasting will be helpful in the success of many endeavors.
Key Concepts in Time Series Forecasting
Trend
The term trend means the long-term movement of a time series data set. It indicates whether the data points tend to increase, decrease, or remain constant. Trends can be straight or bent and take place for short( in months) or long (in decades) periods. Seeing a pattern helps understand why one can appreciate the data, which is essential in making assumptions. For instance, if a company records a consistent increase in its sales for years, it could do better by forecasting the required adjustment in utilizing its stock and marketing actions. Trends make it possible for one to understand future emerging circumstances.
Seasonality
Seasonality is a characteristic of a series in which certain intervals are marked by upturns and downturns that recur with a high degree of regularity. Depending on the data type, these patterns may be noticed daily, weekly, monthly, or yearly. For instance, retail sales tend to increase during the festive seasons or months, such as December, when people purchase gifts for their loved ones. Similarly, electricity usage tends to rise during the hot summer months owing to the use of air conditioning and colder winter months because heating is being used. Recognizing these seasonal trends is critical in ensuring reliable forecasts. However, if understood by the firms, such trends can make changes and inform forecasts and decisions based on the behavioral changes they expect.
Autocorrelation
Stationarity
This research posture gives particular importance to time series data, as its primary statistical characteristics, such as mean, variance, and autocorrelation, which measure how the current value is related to past values, do not change over time. Stationary data is more accessible to analyze and predict as it does not show any underlying trend and seasonal variations. Several forecasting techniques, especially ARIMA, impose that the data must be stationary to yield plausible results. In instances where the time series is not stationary, measures are put in place to ensure that the series is made stationary. One is differencing, which means subtracting the previous and current observations. Detrending is another technique that focuses on eliminating trends from information. Identifying and addressing non-stationary data tendencies is essential when developing effective forecasting models.
Popular Models for Time Series Forecasting
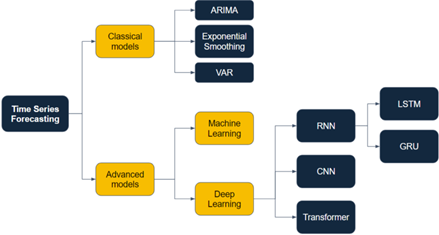
ARIMA (AutoRegressive Integrated Moving Average)
Prophet Model
LSTMs (Long Short-Term Memory Networks)
Time Series Forecasting Applications in Finance
Time Series Forecasting in Economics and Operations
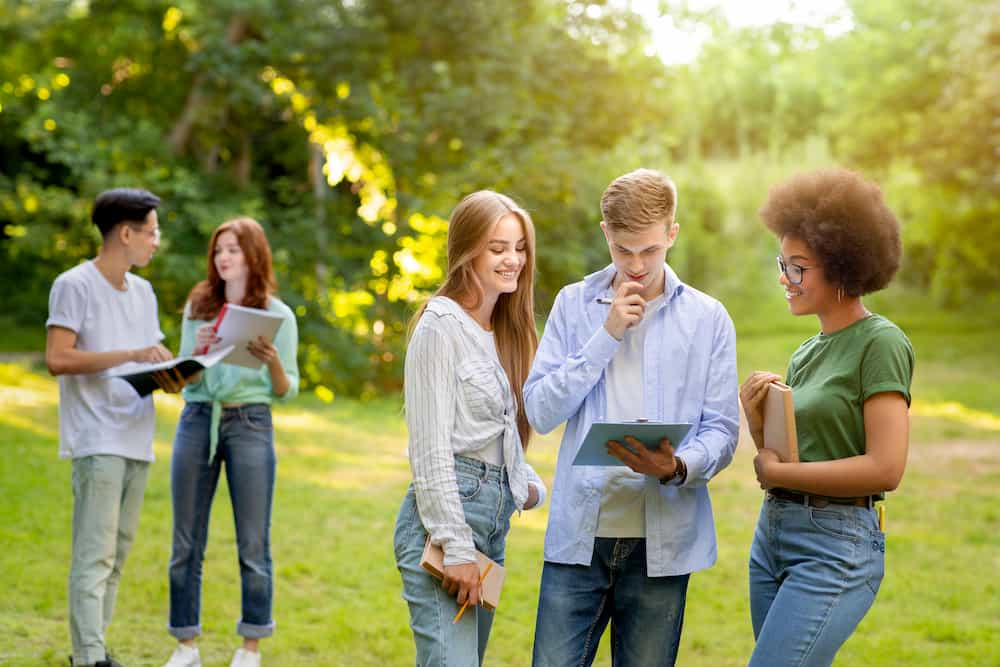