Introduction to Symbolic Systems and Machine Learning:
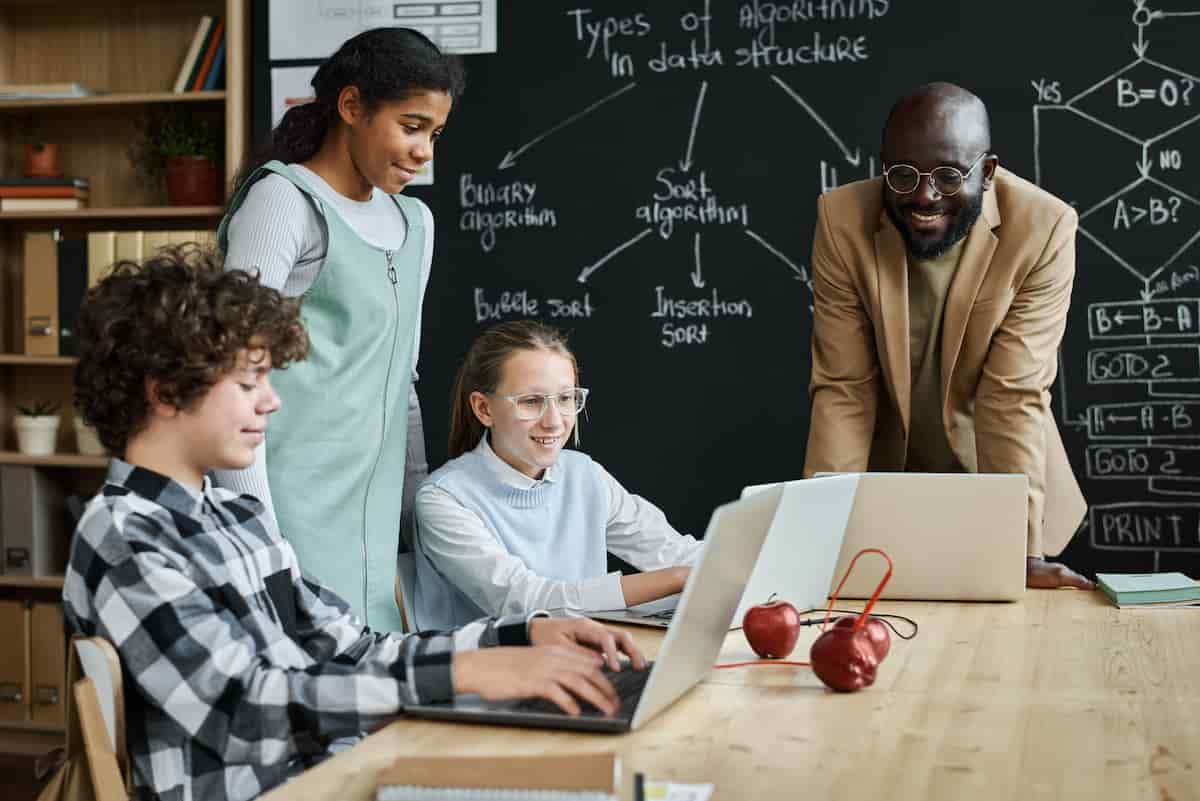
Projects play a crucial role in building a portfolio, serving as tangible evidence of one's skills, experience, and capabilities. As a burgeoning data scientist, one of the most effective ways to demonstrate your skills and knowledge is through hands-on projects. These projects not only illustrate your ability to apply theoretical concepts to real-world problems but also make your portfolio stand out to potential employers. Here are some data science project ideas that can help you boost your portfolio, no matter your skill level.
Projects showcase practical application of theoretical knowledge. While academic achievements and certifications are important, they often do not fully capture an individual's ability to apply what they've learned in real-world scenarios. Projects fill this gap by demonstrating how one can translate their knowledge into action, solving real problems or creating something of value. A well-documented project provides concrete evidence of your experience. Potential employers or clients can see exactly what you have worked on, the challenges you faced, and how you overcame them. This is more compelling than a mere statement of skills or experiences on a resume.
Projects showcase practical application of theoretical knowledge. While academic achievements and certifications are important, they often do not fully capture an individual's ability to apply what they've learned in real-world scenarios. Projects fill this gap by demonstrating how one can translate their knowledge into action, solving real problems or creating something of value. A well-documented project provides concrete evidence of your experience. Potential employers or clients can see exactly what you have worked on, the challenges you faced, and how you overcame them. This is more compelling than a mere statement of skills or experiences on a resume.
Projects often involve encountering and addressing various challenges. By including projects in your portfolio, you can highlight your problem-solving abilities, creativity, and resilience. This is particularly important in fields where innovation and adaptability are key. Through different projects, you can display a range of skills and competencies. For instance, a web developer's portfolio might include projects on front-end design, back-end development, database management, and even cybersecurity measures. This diversity can appeal to a broader audience and open up more opportunities.
A portfolio with detailed projects can build credibility and trust. It shows that you have not only completed tasks but also documented them thoroughly, reflecting professionalism and attention to detail. This can be particularly persuasive for clients and employers who are looking for reliable and thorough professionals. Over time, your projects can reflect your growth and learning. By including a chronological array of projects, you can show how your skills have evolved and how you have taken on increasingly complex challenges. This narrative can be very appealing as it demonstrates continuous improvement and a proactive approach to personal development. Finally, a portfolio with well-documented projects serves as an effective marketing tool. It is something you can present during job interviews, client meetings, or networking events. It provides a structured and visually appealing way to present your professional journey and achievements.
Getting Started with Data Science Projects
Our Office
GREER
South Carolina, 29650,
United States
South Carolina, 29650,
United States