Introduction to Machine Learning Algorithms:
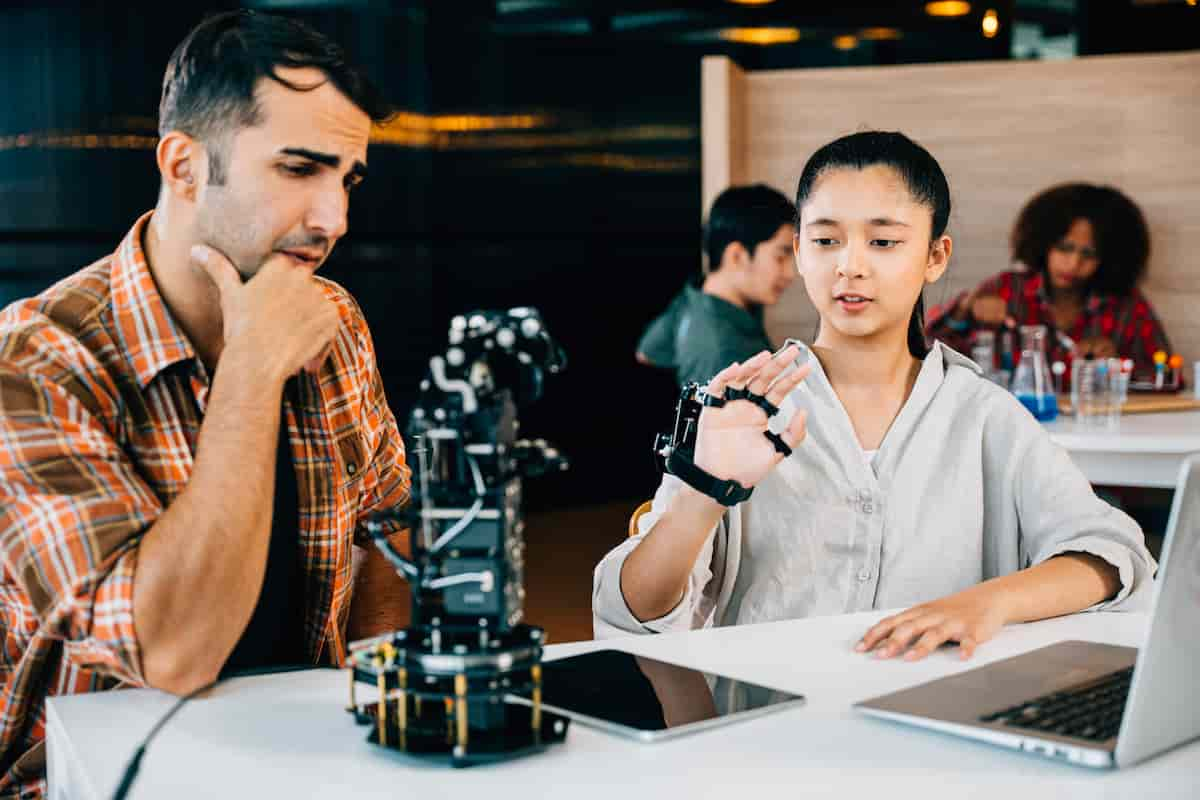
Imagine a computer program that gets smarter with experience, much like a student who learns over time. This is the essence of Machine Learning (ML). Unlike traditional programs, ML algorithms don't rely on explicit instructions for every task. Instead, they learn from data. The more data they encounter, the more accurate they become at making predictions or sorting information. Like a seasoned athlete who adjusts their strategy based on the game, ML continually refines its approach as new data becomes available. This underscores the pivotal role of data in the learning process of ML algorithms, highlighting the significance of data in machine learning. Consider how ML is revolutionizing industries, from predicting stock prices to diagnosing illnesses. It's not just a theoretical concept, but a powerful tool with practical applications.
Demystifying Machine Learning: Its Role in the Modern Era:
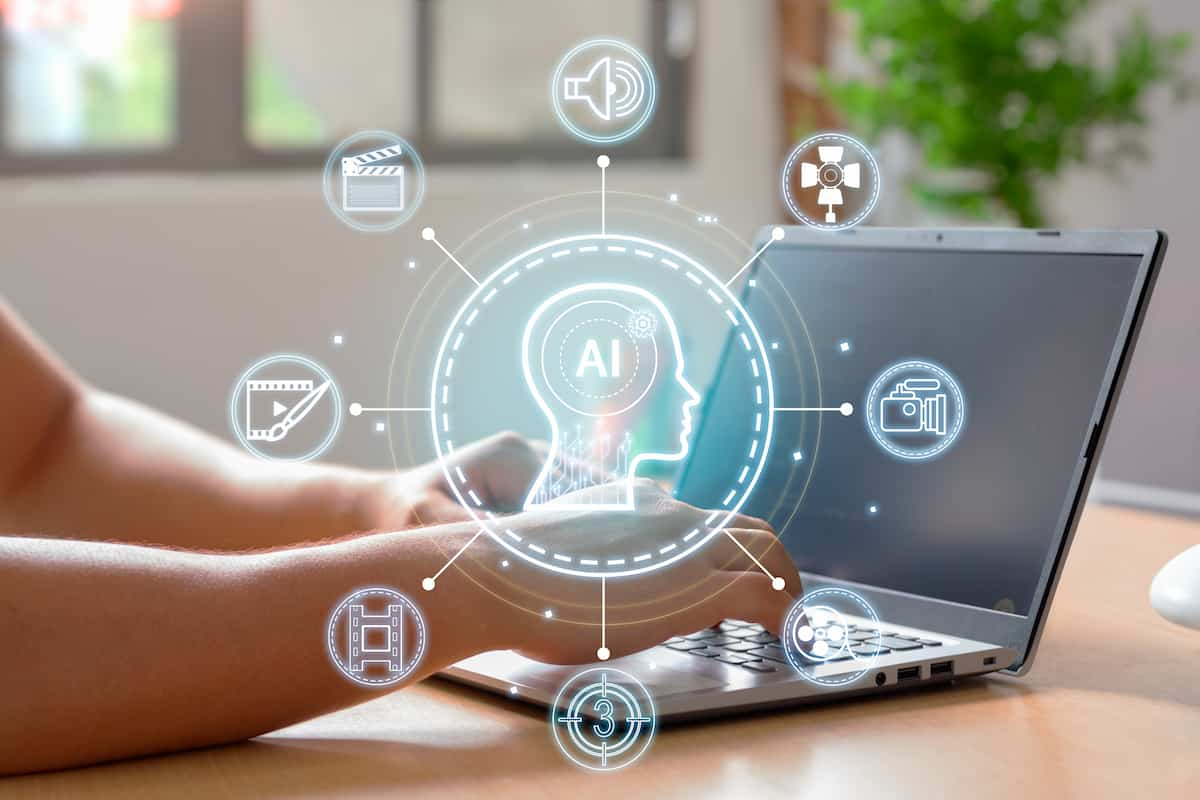
Machine learning is good at finding important stuff in lots of information. This helps people make better decisions about complicated things. Imagine how it's changing things: from guessing stock prices to finding illnesses. It's making industries better and helping people do more.
And there's more! Machine learning makes things easier and faster by doing repetitive jobs for us, like answering customer questions or checking medical pictures rapidly. It's like having a helpful friend who never gets tired. This makes us feel hopeful and sure that we can do great things with machine learning.
Many machine learning algorithms are available, from supervised to unsupervised, reinforcement learning, and more.
These algorithms serve as the bedrock of artificial intelligence (AI) systems, providing the tools to confront real-world complexities head-on. Let's take a closer look at these fundamental building blocks:
Let's dive into these critical programs:
Firstly, supervised learning is a program learned from previously labeled data. This means each piece of information had a tag telling the program what it was. It was great for figuring out things like predicting house prices or
Then, there was unsupervised learning. Here, the programs went exploring data that didn't have labels. They tried to find hidden patterns or groups in the information. They did things like grouping similar stuff or simplifying the data to see what's most important. It helped look at data in new ways, but it was tricky because there were no labels to guide them.
Next up was reinforcement learning. This was like teaching a computer how to play a game by letting it try different things and giving it points when it did well. It was used to train game-playing bots or teach robots to walk or fly. It was great for tasks where you must make decisions step by step.
Finally, there was deep learning, which was a big deal in AI. It used super fancy computer networks called neural networks with many layers to do its job. It was excellent at things like recognizing images or understanding human language. Deep learning was the go-to when you had tons of data and wanted to do cool stuff.
How different algorithms are suitable for various types of problems.
The crucial aspects of performance and efficiency in machine learning algorithms.
Different algorithms foster innovation in machine learning.
Our Office
GREER
South Carolina, 29650,
United States
South Carolina, 29650,
United States