Integrating cutting-edge technologies is crucial for staying competitive in the manufacturing domain. One such technology making waves is machine learning (ML). This article explores the diverse applications of machine learning in manufacturing, showcasing its potential to transform traditional processes into efficient and intelligent operations.
Introduction:
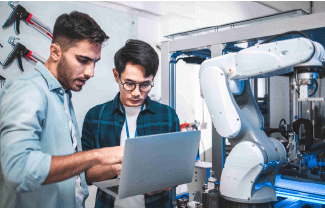
Evolution of Manufacturing:
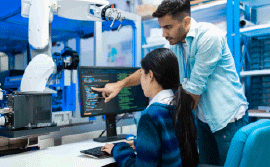
Smart Manufacturing and Machine Learning:
Goals of Smart Manufacturing:
- Smart Manufacturing is guided by several key objectives, as outlined in the paper above:
a. Automation for Maximized Efficiency: Machine learning is pivotal in automating manufacturing processes, leading to unparalleled levels of efficiency.
b. Sustainability Enhancement: Integrating machine learning contributes to increased sustainability, aligning manufacturing practices with environmental consciousness.
c. Efficient Supply Chain Management: Smart Manufacturing seeks to optimize supply chain management through intelligent data processing.
d. Proactive System Barrier Identification: Machine learning aids in identifying potential system barriers before they manifest, enabling preemptive problem-solving.
Goals of Smart Manufacturing:
Improved Assembly Line Efficiency: Harnessing machine learning to optimize assembly line processes, enhance efficiency, and reduce operational bottlenecks.
- Improved Customer Efficiency (Personalization): Utilizing AI to personalize customer experiences, tailoring products and services to individual preferences for enhanced satisfaction.
- Optimized Inventory Management: Applying machine learning algorithms for precise inventory management, minimizing waste, and ensuring optimal stock levels. Real-Time Insights and Inventory Visibility: Leveraging AI to provide real-time insights into manufacturing processes and ensuring visibility into inventory levels. Delivery Route Optimization: Using machine learning for intelligent route planning, minimizing transportation costs, and optimizing delivery schedules.
- Minimizing Loss Associated with Delayed and Damaged Goods: Implementing AI to mitigate losses by identifying and addressing issues related to delayed or damaged goods in real-time.
- Product Design and Development: Integrating simulations-based product design and manufacturing decisions accelerates innovation and development. They are employing machine learning to gather and analyze customer requirements, facilitating the creation of tailored products and solutions, and leveraging AI to explore and generate novel design concepts, fostering innovation in product development.
- Predictive Maintenance (One of the Hottest Areas in Smart Manufacturing): Embracing predictive maintenance powered by machine learning to identify and detect potential machine failures before they impact production.
- Increasing Supply Chain Visibility: Using AI to enhance supply chain visibility enables better tracking and management of resources throughout the supply chain.
Computer Vision: Revolutionizing Part Inspection and Process Monitoring
At the forefront of machine learning's impact in manufacturing lies the domain of computer vision-based part inspection. Manufacturers can achieve high-throughput part inspection by harnessing cost-effective sensors, particularly RGB cameras empowered with machine learning algorithms. This symbiotic relationship between advanced sensors and machine learning algorithms enhances the speed of inspections and ensures a meticulous and accurate analysis of each component.
Precision and Efficiency: Machine Fault Detection and Prognostics in Manufacturing
Harnessing Machine Learning for Process Optimization in Manufacturing
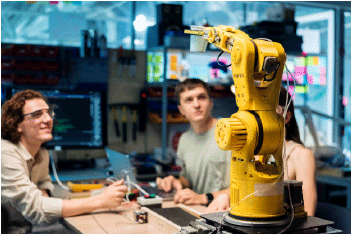
Conclusion:
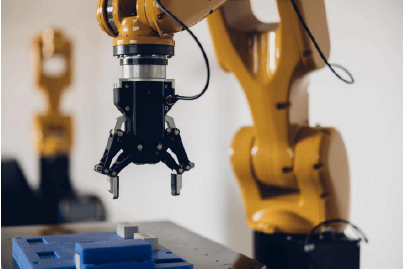
FAQs
- What is the significance of machine learning in manufacturing?
Machine learning in manufacturing is pivotal for optimizing processes, reducing downtime, and enhancing efficiency. It enables timely fault detection, process monitoring, and predictive maintenance, contributing to a more streamlined and competitive manufacturing environment. - How does computer vision impact part inspection in manufacturing?
Computer vision transforms part inspection by leveraging AI algorithms and cost-effective sensors. This allows for high-throughput inspection, ensuring accuracy and efficiency in identifying and addressing potential defects, thus improving overall manufacturing quality. - What role does AI play in supply chain optimization for manufacturing?
AI optimizes supply chain management by analyzing vast datasets, improving inventory management, and enhancing visibility. This results in a streamlined, efficient supply chain that adapts quickly to changes and minimizes disruptions. How can machine fault detection reduce downtime in manufacturing?
Machine fault detection identifies potential issues before they escalate, allowing for proactive intervention. This reduces machine downtimes, ensuring continuous production and providing a strategic advantage for manufacturing companies.What is the intersection of machine learning and process optimization in manufacturing?
Machine learning tools create input-to-output mapping functions, optimizing manufacturing processes. This synergy enables faster mass production, customization, and minimizes waste, contributing to efficient and agile manufacturing practices.How does AI impact product design and development in manufacturing?
AI facilitates simulations-based product design, aiding in the generation of novel concepts and accelerating the innovation process. It enhances the efficiency and creativity of product development in the manufacturing sector.What are the applications of AI and ML in smart manufacturing?
AI and ML applications in smart manufacturing include automation of processes, improved sustainability, efficient supply chain management, and real-time insights into manufacturing operations.How does machine learning contribute to quality control in manufacturing?
Machine learning is instrumental in quality control by detecting defects, ensuring product consistency, and minimizing errors. It enhances overall product quality and reliability in manufacturing.What are the key benefits of applying AI in the manufacturing domain?
The key benefits include increased efficiency, reduced downtime, enhanced product quality, streamlined processes, and the ability to adapt quickly to changing demands. AI empowers manufacturing companies to stay competitive and innovative in the dynamic market landscape.How does AI contribute to the continuous monitoring of manufacturing processes?
AI contributes to continuous monitoring by integrating computer vision and machine learning. This integration provides instantaneous visibility across all stages of the manufacturing process, spanning from assembly to the final product. It ensures the early identification of potential issues, allowing for proactive interventions and enabling data-driven decision-making to enhance overall operational efficiency.
Our Office
South Carolina, 29650,
United States