Shifting Gears from Gaming to AI: Nvidia's Strategic Transformation
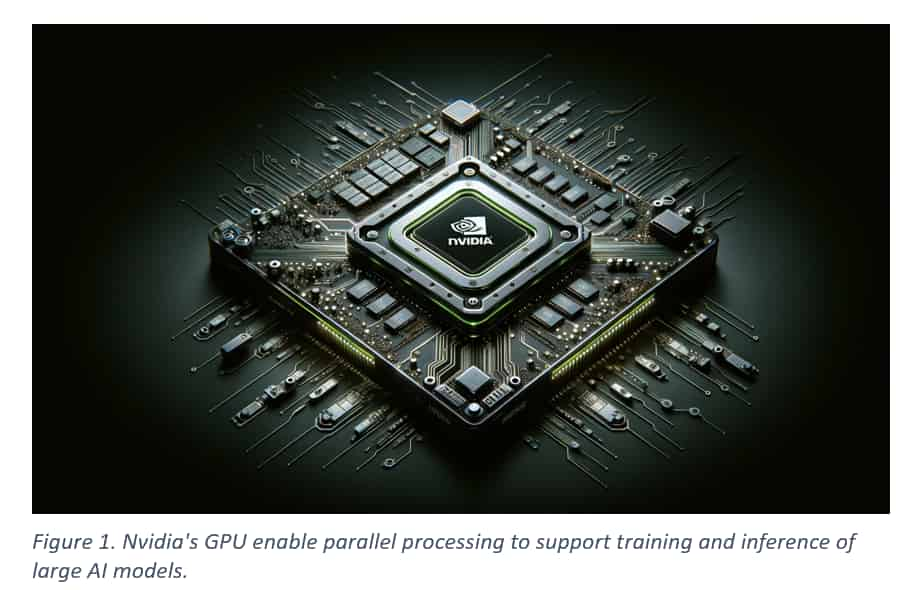
Why GPUs are Essential for AI Computations?
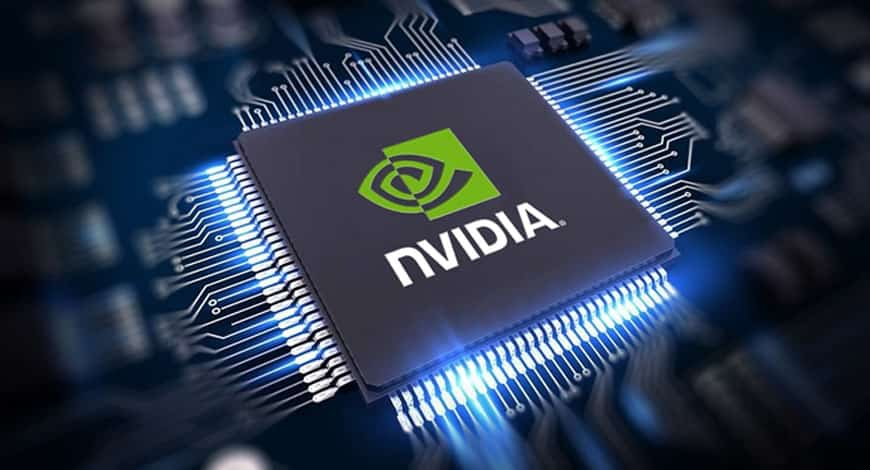
Nvidia vs. The Competition
Nvidia’s Ecosystem: Collaborations and Innovations in the AI space
Why Nvidia Matters in AI’s Next Chapter
FAQs
- What is a GPU and why is it important for AI?
A GPU, or Graphics Processing Unit, is a specialized electronic circuit designed to rapidly manipulate and alter memory to accelerate the creation of images in a frame buffer intended for output to a display device. For AI, GPUs are crucial because they can perform multiple calculations simultaneously, making them highly efficient for the parallel processing required in machine learning and deep learning tasks. - How does Nvidia compare to its competitors in the AI chip market?
Nvidia is considered a leader in the AI chip market, primarily due to its advanced GPU technology, comprehensive software ecosystem, and strategic partnerships. While competitors like Intel, AMD, and Google offer their versions of AI-optimized hardware, Nvidia's GPUs are widely adopted for their superior performance in parallel processing and deep learning applications. - What are Tensor Cores?
Tensor Cores are specialized hardware found in Nvidia GPUs designed to accelerate deep learning tasks. They are optimized to perform tensor operations, which are fundamental in neural network computations, offering higher efficiency and speed for AI model training and inference. What is CUDA?
CUDA (Compute Unified Device Architecture) is a parallel computing platform and programming model developed by Nvidia for general computing on its own GPUs. It allows developers to use GPUs for computational tasks traditionally handled by CPUs, significantly improving performance in applications like machine learning, scientific simulations, and 3D rendering.Why are GPUs considered more efficient than CPUs for AI tasks?
GPUs are designed for parallel processing, meaning they can handle multiple operations at the same time. This is particularly useful for AI tasks that involve processing vast amounts of data simultaneously. CPUs, while versatile, are optimized for sequential processing and cannot match the speed and efficiency of GPUs for specific AI computations.What role does Nvidia's software ecosystem play in AI development?
Nvidia's software ecosystem, including CUDA, cuDNN, and TensorRT, provides developers with powerful tools and libraries to optimize AI applications for GPU computing. This ecosystem enables efficient programming, faster execution of AI models, and support for a wide range of AI and machine learning frameworks, making it easier for developers to build and deploy AI solutions.How does Nvidia's technology contribute to real-world AI applications?
Nvidia's GPUs and AI technologies are used in a wide range of applications, from autonomous vehicles and healthcare diagnostics to voice recognition and financial modeling. By providing the computational power necessary for complex AI calculations, Nvidia's technology enables faster, more accurate results in these applications.What is the significance of Nvidia's collaborations in the AI space?
Nvidia collaborates with academic institutions, industry leaders, and cloud providers to advance AI research, develop new AI technologies, and make AI more accessible. These collaborations help drive innovation, foster the adoption of AI across various sectors, and ensure that Nvidia's technologies are integrated into leading AI applications and platforms.How is Nvidia addressing the challenges of energy efficiency in AI computations?
Nvidia is focused on designing GPUs that not only deliver high performance but also operate efficiently to reduce energy consumption. The company invests in research and development to improve the energy efficiency of its chips and is exploring ways to minimize the environmental impact of AI computations.What future developments can we expect from Nvidia in the AI field?
Nvidia continues to push the boundaries of AI technology with ongoing research into more powerful and efficient GPUs, advancements in AI software, and initiatives to expand the AI ecosystem. Future developments may include new chip architectures, enhanced AI programming models, and collaborations aimed at solving some of the most pressing challenges in AI and computing.
Our Office
South Carolina, 29650,
United States