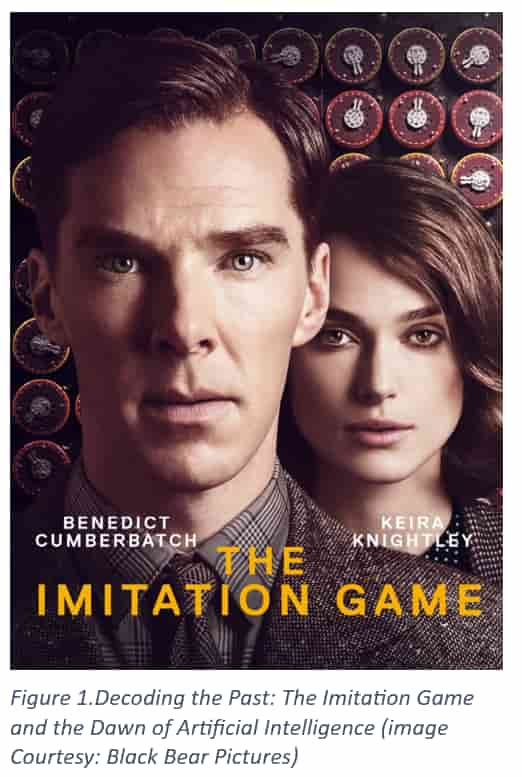
Milestones in AI: From Chessboards to Chatbots
As the curtain rises on the stage of artificial intelligence, we are greeted by the visage of a historic chessboard where Garry Kasparov, the grandmaster, contemplates his next move against Deep Blue. In 1997, this supercomputer, a marvel of its time, did the unthinkable—it defeated Kasparov, signaling to the world that machines could not only calculate but strategize, outmaneuver, and outthink human minds in one of our oldest intellectual games. Fast forward a decade to 2007, and we find ourselves navigating the urban labyrinths with unprecedented ease, thanks to Google Maps. With its intricate AI-based search algorithms and real-time data processing, it transformed trip planning from an exercise in cartography to a few simple taps on a screen. The technology's influence expanded beyond mere convenience; it became a foundational component in the burgeoning field of location-based services.
The journey through AI's milestones brings us to the bright lights of a Jeopardy studio in 2011, where IBM's Watson stands—not merely a computer, but a contestant. Watson's victory over trivia titans, including the legendary Ken Jennings, demonstrated machine learning's potential to comprehend, process, and respond to natural language with precision—a formidable leap toward AI systems that could understand and interact with us on a human level. In the penultimate scene of our exploration, we encounter ChatGPT, which was released in November 2022. ChatGPT offered a new frontier of interaction, showcasing an AI's ability to engage in dialogue, generate text, and offer insights with an uncanny resemblance to humans.
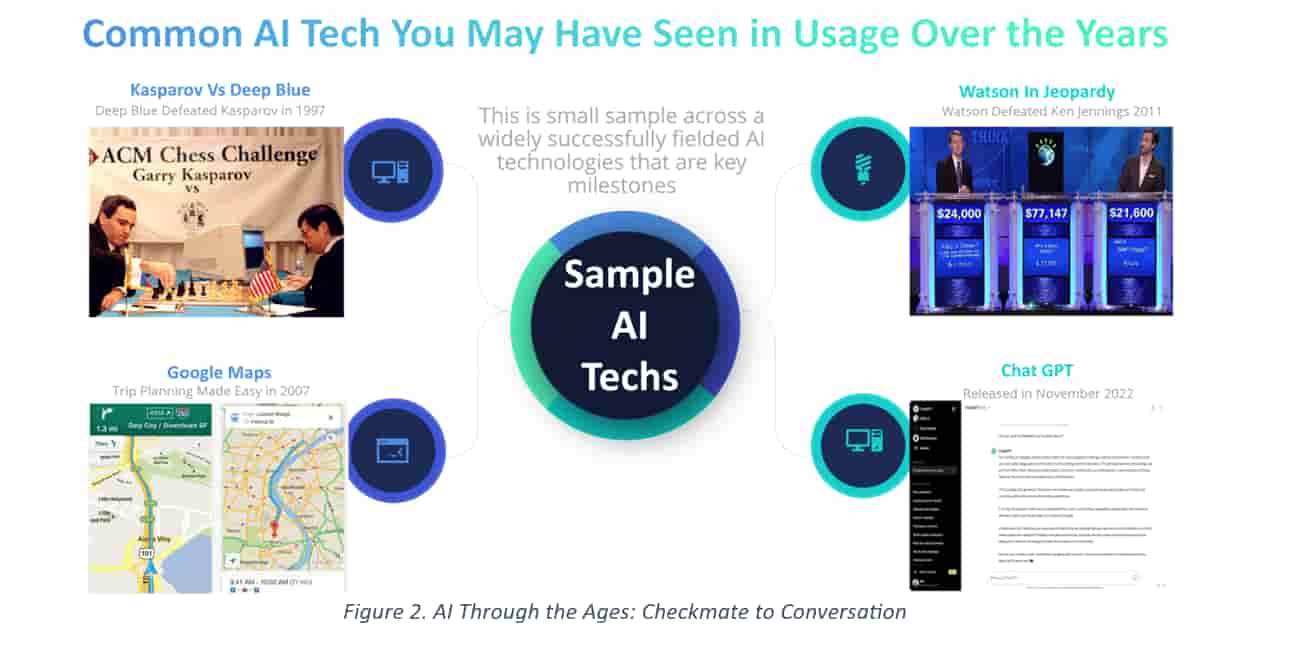
The real impact of AI's evolution is perhaps most vividly illustrated in the realms of marketing and IT within leading tech behemoths like Google, Facebook, and Amazon. Google's algorithms have mastered the art of search engine marketing, leveraging AI to deliver tailored advertisements at an individual level, effectively transforming clicks into revenue with precision-targeted campaigns. Facebook, the social media juggernaut, uses advanced AI to understand user preferences, customize content delivery, and safeguard platforms against fraudulent activities. Amazon's recommendation engines, powered by AI, have revolutionized the shopping experience, predicted customer desires and effectively placing products into the virtual hands of consumers.
In the last two decades, the impact of AI on IT and digital marketing sectors has been profound, marking a shift from traditional methods to dynamic, data-driven approaches that continually learn and improve. AI has redefined the landscape of consumer engagement, operational efficiency, and market competition, setting a new standard for what is possible. As we stand on the shoulders of these AI giants, looking out onto the horizon of what's to come, it is essential to appreciate the journey that has brought us here. Understanding the historical development of AI provides context for current advancements. It allows individuals to grasp how AI evolved from its early stages to the sophisticated technologies we have today, enabling us to appreciate the depth of its influence and to anticipate the waves of change that the future holds.
AI Evolution: A Journey Through Decades of Innovation
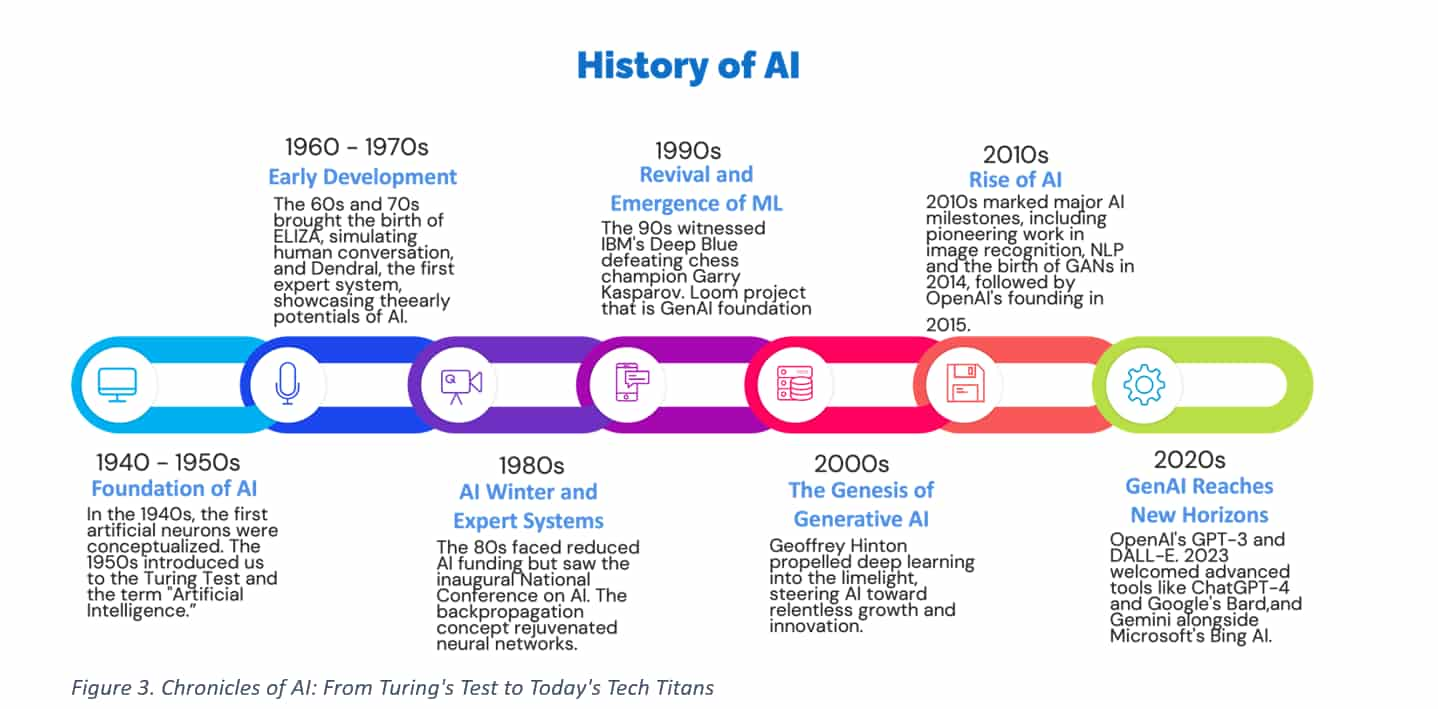
Dawn of Digital Thought: The Pioneering AI Era of the 1940s-1950s
Then came 1956, a watershed moment at the Dartmouth Conference, which not only birthed the term "Artificial Intelligence" but also brought together minds like Marvin Minsky, Claude Shannon, and Nathaniel Rochester. This gathering cemented the status of AI as a distinct academic discipline and established a daring objective: to unravel the means by which machines might utilize language, forge abstract ideas, tackle problems hitherto exclusive to human reasoning, and enhance their own capabilities. Beyond these notable events, the 1950s also saw the birth of the "Logic Theorist" byAllen Newell andHerbert A. Simon, which became known as the first AI program. The intent of the program was to prove mathematical theorems, heralding the era of machines performing tasks that required human-like intelligence. As the decade closed, the stage was set for the ensuing growth of AI. The culmination of these developments was not just technological advancement but also a philosophical introspection about the essence of human intellect and the boundless potential of machines.
AI Ascendant: Bridging Human and Machine Intelligence, 1960s-1970s
AI Winter to Renaissance: Navigating the 1980s in Artificial Intelligence
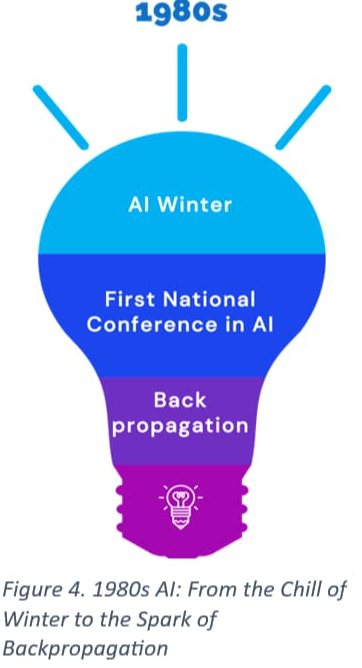
AI Breakthroughs of the 1990s: Pioneering Machine Learning and Historic Chess Matches
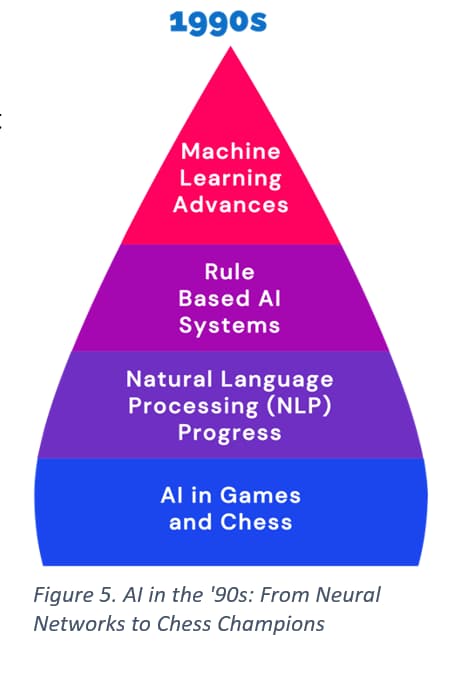
The era was also notable for the continued reliance on and sophistication of rule-based AI systems. These systems, built upon a foundation of predefined rules and expert knowledge, were instrumental in the development of expert systems that could diagnose diseases, offer financial advice, and even predict mechanical failures.
In the natural language processing (NLP) realm, significant strides were made. Innovations in this decade laid the groundwork for complex applications such as machine translation, text summarization, and early virtual assistants. These advancements allowed for more nuanced understanding and generation of human language by computers, a precursor to the sophisticated chatbots and voice-activated assistants we see today.
One of the most publicized AI milestones of the 1990s was IBM's Deep Blue chess program defeating world champion Garry Kasparov in 1997. This was not just a victory on the chessboard; it symbolized the potential of AI to handle complex, strategic decision-making processes, a feat that was believed previously to be the exclusive of human intellect.
The 1990s also saw the growth of the internet and the beginning of the 'dot-com' boom, which provided a new platform for AI applications to proliferate. Search engines began employing AI to better index and rank web pages, while e-commerce sites started using recommendation systems to personalize user experiences. As the decade closed, AI was on the cusp of a new era, fueled by increased computational power, the proliferation of data, and a renewed interest from both academia and industry. The accomplishments of the 1990s solidified AI's place in the world, not as a passing fad, but as a field ripe with endless possibilities, set to revolutionize every aspect of human life.
2000s: The Big Data Boom and the Deep Learning Revolution
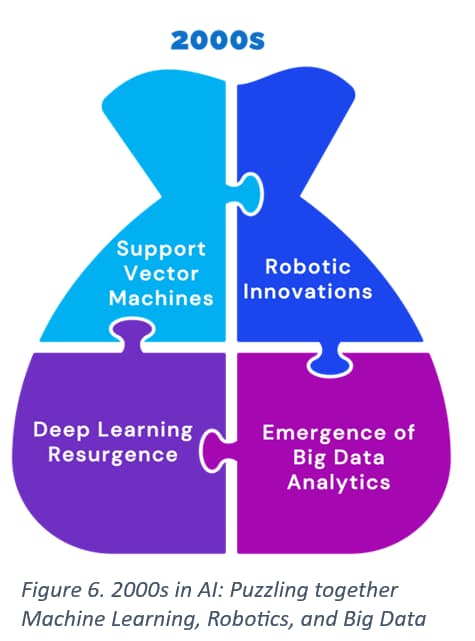
The evolution of decision tree branched out further with the introduction of the Random Forest algorithm by Leo Breiman in 2001. This ensemble learning technique combined the simplicity of decision trees with the power of diversity, creating a forest of trees where each tree's decision contributes to a more accurate and robust consensus. Nestled within the expansive forest of machine learning methodologies, decision trees stand out for their intuitive approach to decision-making, tracing their roots back to the earliest days of AI. The 1960s saw the genesis of decision tree algorithms, but it wasn't until the 1980s that they were refined and popularized by researchers likeRoss Quinlan, who developed the ID3 algorithm in 1986 and later the C4.5 in 1993, which became standards for machine learning decision tree classifiers. These algorithms reinforced the notion that sometimes, a collective decision-making process can lead to stronger, more reliable outcomes—a concept that mirrors the very essence of human societal structures.
2010s: The Decade AI Mastered Language, Games, and Generative Arts
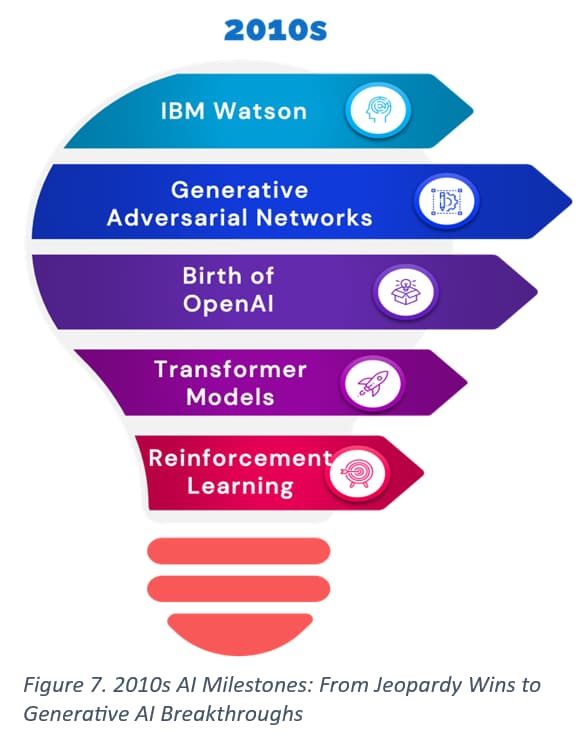
By the end of the 2010s, AI was not a distant scientific dream but a real and present part of our daily lives. From transforming healthcare diagnostics to powering personal assistants and driving autonomous vehicles, the 2010s will be remembered as the decade when AI ceased to be just a subject of science fiction and became a vital part of the human story.
2020s: The Generative AI Era
Going Forward: Three Major Future Directions of AI
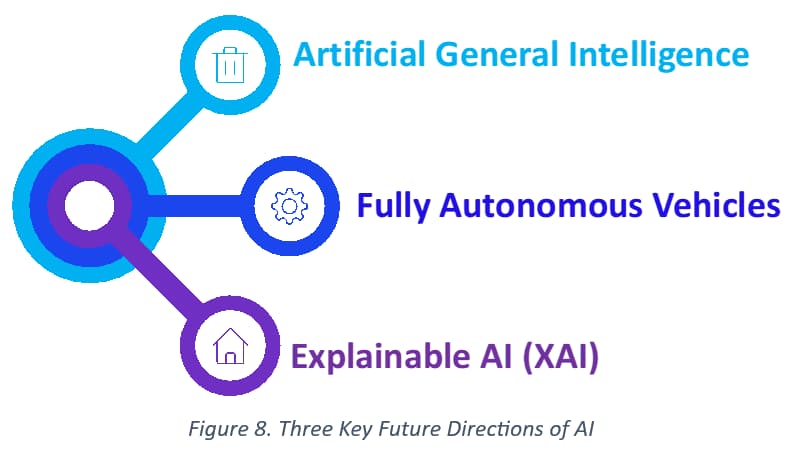
Our Office
South Carolina, 29650,
United States