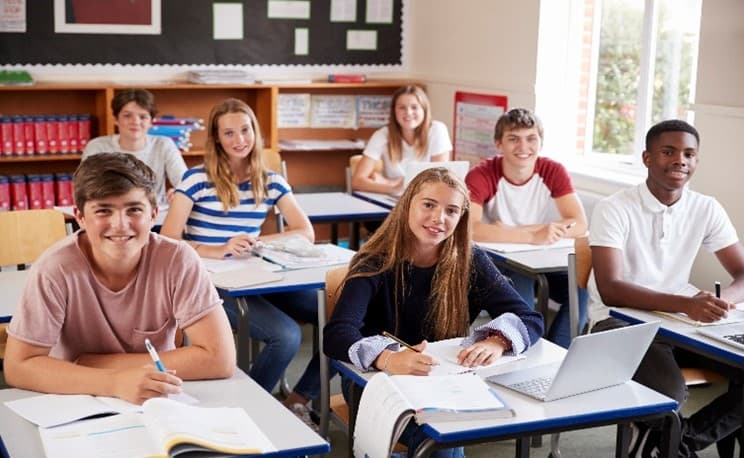
As the world continues to generate an enormous amount of data, it is becoming increasingly apparent that learning how to code will be essential for all students, especially those who wish to study something such as data science. This trend has now crossed over to high schools, where students are tasked with data science projects to tackle real-life issues, appreciate data's relevance, and sharpen their critical thinking abilities.
Why is coding important in data science?
Writing code is critical because it empowers students to work smart with data. Handling data on a human scale is relatively slow, error-prone, and highly restricted, especially when handling large amounts of data. For instance, consider a learner who wants to calculate the test scores of countless students from various schools, ages, genders, and socioeconomic backgrounds and their study behavior. To put it mildly, such a task would be challenging, if not impossible, without coding. With coding, students can take it up by finding ways to eliminate task redundancy, conduct data analysis efficiently and accurately, and even present the findings.
Learning how to code also trains students to develop a particular way of thinking in a structured manner. Depending on the assignment they are working on, students may need to simplify the task into various steps, compose a set of commands for a machine to execute, and check that the operations have been performed accurately. Such degrees of logical reasoning come in handy for data science and enhance their problem-solving ability. Additionally, coding is an ability that can be applied in other areas; once a student understands the concept of coding, it ceases to be relevant to data science and includes app development, machine learning, and game design, among other fields. The potential to code presents many opportunities and supports the development of creative thoughts throughout one’s life.
What programming languages should high school students learn for data science?
Two programming languages are overused in data science: Python and R. These languages are embraced by the industry and scholars alike due to their flexibility, ease of use, and the many tools available for working with data.
For many high school students inclined towards pursuing studies in data science, one language will most likely be introduced to them in their very early stages: Python. It is apparent why Python will be the first language for students learning data science. Python is simple and easy to read, encouraging people who are timid about coding, especially novices. There is also a vast population of users, meaning that students will have access to numerous tutorials, guides, and forums where help can be sourced. Beyond its accessibility, Python boasts powerful libraries specifically designed for data manipulation, analysis, and visualization. Thanks to libraries like Pandas for data manipulation and Matplotlib and Seaborn for data visualization, students can load, clean, and transform data — in no time. In addition, Python is a multipurpose programming language that students can use outside their data science projects, such as web development, automation, etc., or even Artificial Intelligence (AI).
On the other hand, R was made with the only goal of doing statistical analysis and data visualization; hence, it is very focused and task-oriented regarding data science. If R can be said to appeal to students more with excess maths and statistics training than training on data itself, then it is a more than helpful tool for data. Its libraries include but are not limited to, dplyr for data manipulation and ggplot2 for visualization, enabling students to carry out advanced statistical analysis and produce highly polished and flexibly structured graphics. R enjoys high popularity and penetration into academic and research institutions where correct statistics and complex data modeling are paramount.
The selection of programming languages, R and Python, pivots on the background and aspirations of the student. Students aiming at a more interdisciplinary approach may find a more significant appeal in this general-purpose, constantly evolving programming language. In contrast, R may better suit students interested in statistics and pure data science. However, both languages provide high school students the tools to take their data science projects to the next level.
Coding in Data Science: The Essential Skills
Data Manipulation
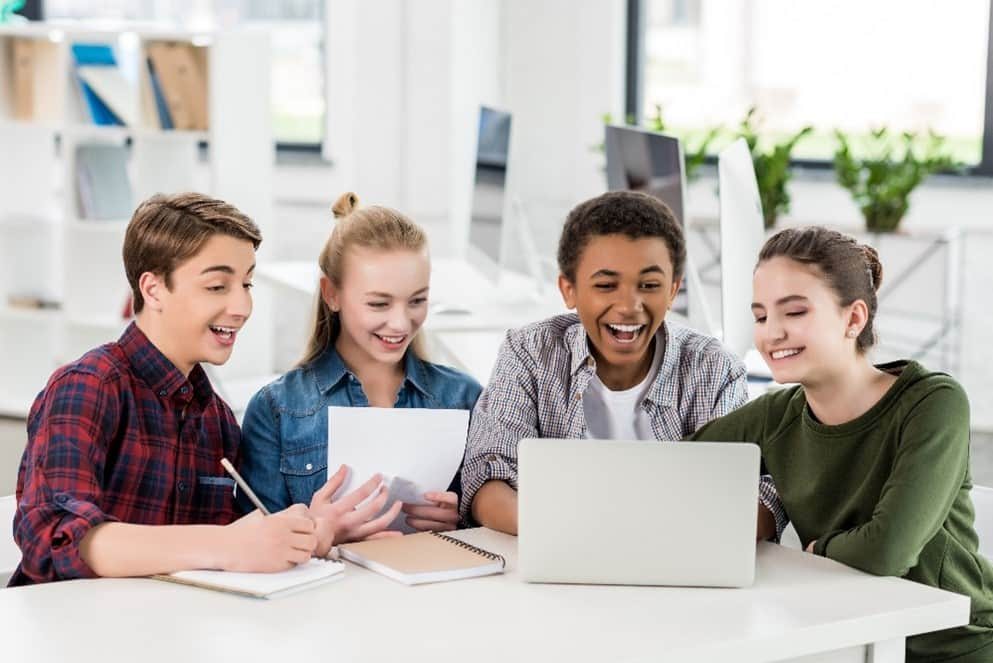