Chatbots: Enhancing Conversations with AI
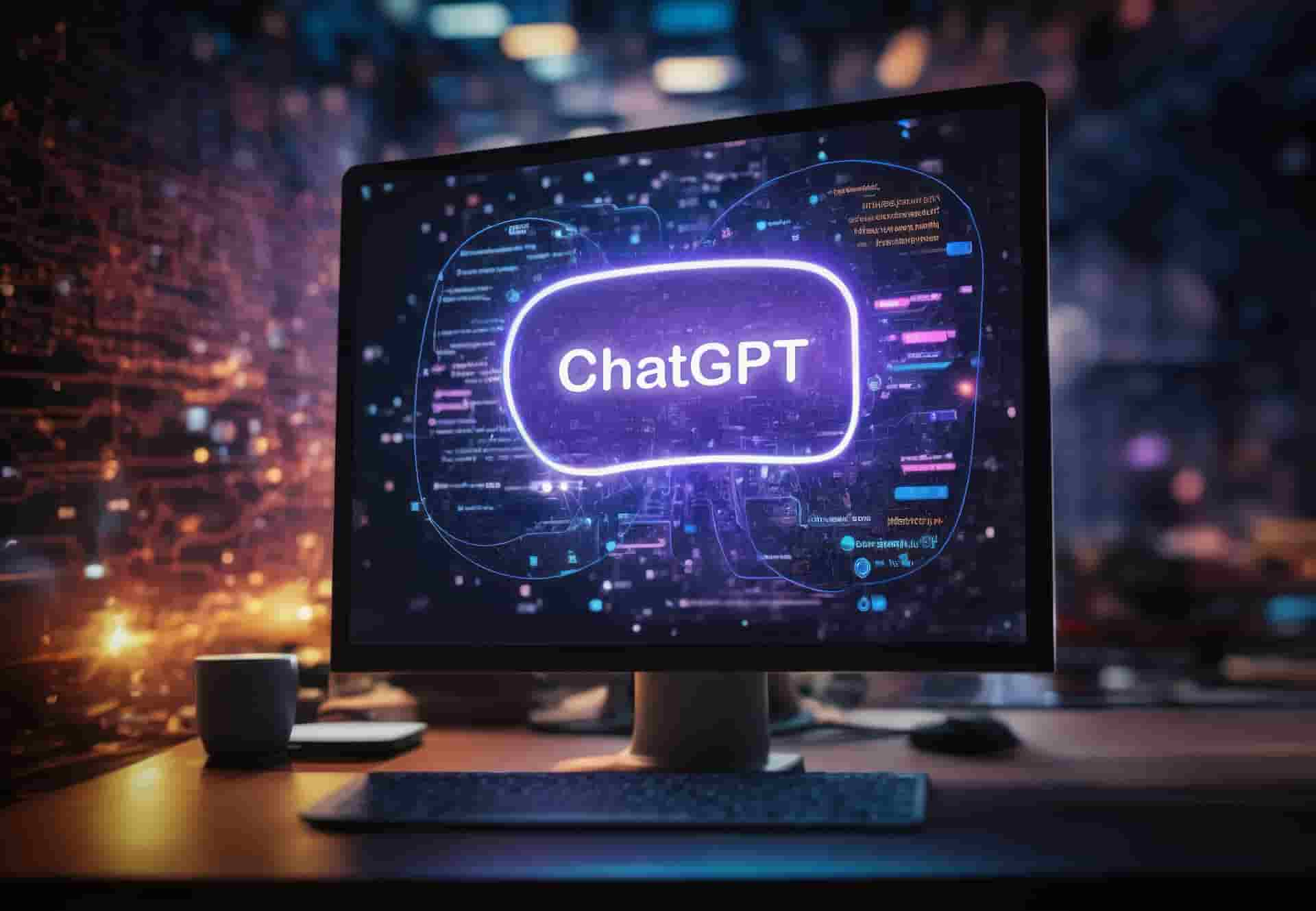
What is a Chatbot?
A chatbot is a software application crafted to mimic human conversation. It interacts with users through text or voice, providing automated responses. While not all chatbots possess artificial intelligence (AI), modern ones increasingly leverage conversational AI techniques, such as natural language processing (NLP), to understand user queries and generate relevant answers.
The Purpose of Chatbots
Chatbots serve diverse purposes across industries, revolutionizing communication and efficiency. Here are some key roles they play:
Customer Service and Support:
A chatbot is a software application crafted to mimic human conversation. It interacts with users through text or voice, providing automated responses. While not all chatbots possess artificial intelligence (AI), modern ones increasingly leverage conversational AI techniques, such as natural language processing (NLP), to understand user queries and generate relevant answers.
The Purpose of Chatbots
Chatbots serve diverse purposes across industries, revolutionizing communication and efficiency. Here are some key roles they play:
Customer Service and Support:
- Chatbots offer 24/7 assistance, promptly addressing customer queries and resolving issues.
- They handle routine tasks like tracking orders, resetting passwords, and providing product information.
- By automating responses, chatbots reduce the need for human intervention, improving efficiency and cost-effectiveness.
Lead Generation and Sales:
- Businesses use chatbots to engage potential customers.
- Chatbots can guide visitors through a preset questionnaire, encouraging lead generation and boosting conversion rates.
- They provide instant information, nurturing leads and driving sales.
Internal Processes and Productivity:
- Within organizations, chatbots streamline processes.
- They assist employees by answering HR-related questions, managing leave requests, and providing company information.
- Chatbots automate routine tasks to free up human resources for more strategic work.
Enhancing User Experience:
- Chatbots keep users engaged on websites and apps by providing quick answers.
- Chatbots keep users engaged on websites and apps by providing quick answers.
Personal Assistants and Task Automation:
- Chatbots act as virtual personal assistants, scheduling meetings, setting reminders, and organizing tasks.
- They integrate with tools like calendars and to-do lists, enhancing productivity.
Industries Leveraging Chatbots
Our Office
GREER
South Carolina, 29650,
United States
South Carolina, 29650,
United States