Key Takeaways:
- Exploring successful partnerships between colleges, nonprofits, for-profit ed-tech companies, and high schools is pivotal for supporting, implementing, and sustaining high-quality K-12 data science programs. These alliances form the backbone of a collaborative approach to data science education, ensuring a seamless integration from high school to higher education.
- The K-12 data science curriculum needs to bridge abstract data science concepts to real-world applications that are relatable to K-12 high school students. Educators can break away from traditional teaching methods by adopting innovative approaches such as hybrid learning methodologies, making data science fascinating and accessible for K 12 students.
- Recognizing the variability in teacher preparedness is crucial in navigating the challenges of data science education in K-12. From the scarcity of relevant datasets to the imperative need for teacher training, the availability of good online courses addressing these challenges becomes a stepping stone towards a future where every student has the opportunity to engage meaningfully with data science. Supporting teachers through targeted professional development programs, resources, and mentorship opportunities is essential to create a unified and impactful educational experience.
Introduction:
Part 1: Bridging the Gap - Successful Partnerships in Data Science Education
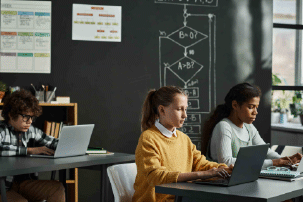
- College Collaborations: Establishing partnerships between local high schools and colleges can bring a wealth of expertise and resources. Colleges can provide curriculum development support, access to experienced instructors, and exposure to real-world data science applications and create summer programs for high school students. This collaboration makes a seamless bridge between high school and higher education. Example programs: Stanford University Data Science for High School (DSHS), University of California, Berkeley - Foundations of Data Science, and Carnegie Mellon University Pre-College Programs - AI Scholars program.
- Nonprofit Initiatives: Nonprofits dedicated to education play a pivotal role in filling gaps within the system. These organizations can offer funding, mentorship programs, and curriculum resources by teaming up with high schools. They can act as catalysts for change, driving the integration of data science education into the K-12 curriculum. Example Programs: Data Science for Social Good (DSSG), Data Science 4 everyone, and DataKind.
- Industry Involvement: Bringing industry players into the equation ensures students receive a practical and relevant education. Partnerships with tech companies and data-driven industries can lead to internships, guest lectures, and exposure to cutting-edge technologies. This enhances the learning experience and prepares students for the demands of the real world. Example Programs: IBM Machine Learning for Kids, and Microsoft- Imagine Cup Junior.
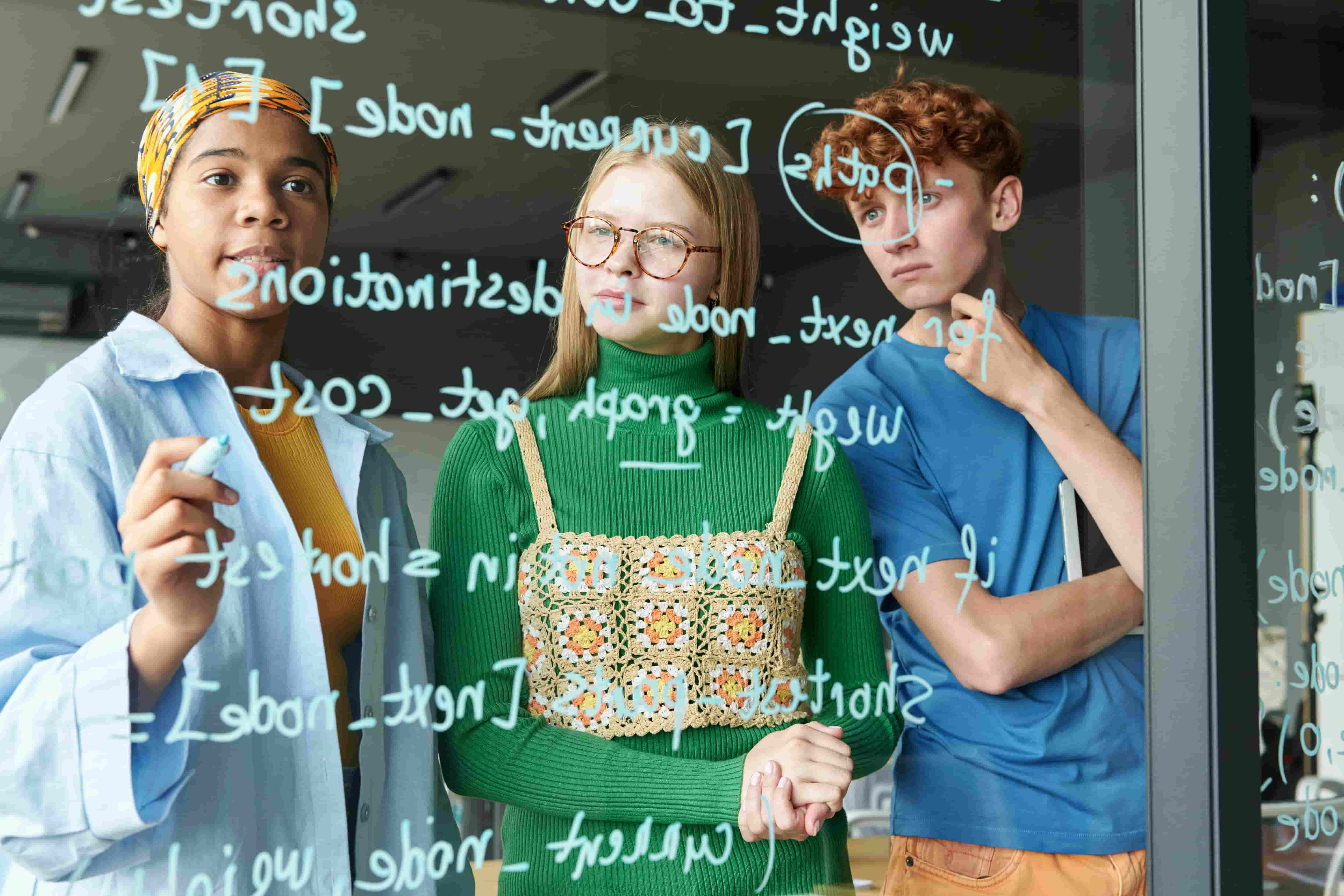
- For-profit K-12 ed-tech companies can develop interactive and user-friendly learning platforms specifically designed for K-12 students. These platforms should incorporate gamified elements, hands-on projects, and real-world applications to make data science concepts engaging and accessible. Implementing adaptive and personalized learning technologies that cater to individual student needs can also be enabled by for-profit ed-tech organizations. Customized learning experiences can help students progress at their own pace, ensuring that each student receives the support and challenges appropriate for their skill level. NextGen BootCamp, AIBrilliance Online Hybrid Bootcamp, and CodeHS Data Science with Python.
- Teacher Training Programs: High-quality professional development is essential for effectively delivering data science education. Partnerships focusing on training educators in the latest tools, methodologies, and best practices ensure that students receive instruction from well-prepared and informed teachers. Data Science Education Community of Practice (DSECOP).
- Community Engagement: Collaboration with the local community can foster a supportive environment for data science education. Initiatives such as hackathons, data challenges, and community projects create opportunities for K-12 students to apply their knowledge in real-life scenarios, making learning more engaging and impactful.
By joining forces, colleges, nonprofits, and high schools can collectively shape a future where data science is not just a skill but an integral part of every student's education. Together, we can bridge the gap and empower the next generation with the tools they need to thrive in a data-driven world.
Part 2: Making Data Science Tangible - Engaging Students in the Classroom
Title: Breaking Barriers: Making Data Science Tangible for Students
In K-12 education, connecting abstract concepts like data science to real-world applications can often pose a significant challenge. This challenge becomes particularly pronounced when it comes to keeping students engaged. Many science teachers find themselves grappling with the struggle to captivate their students, resorting to conventional methods like slides, lectures, and traditional science experiments to collect data.
The traditional approach, while familiar, can be time-consuming to set up and maintain. Teachers, in their role as educators, are pressed for time and resources. This limitation not only impacts their ability to delve deeper into the subject matter but also affects the overall engagement of students. Teachers need the space and time to be true educators, fostering an environment that nurtures curiosity and exploration. The key lies in breaking away from the monotony of conventional teaching methods and embracing innovative approaches, such as hybrid learning (recorded online lectures + hands-on in-person activities), that make data science an abstract concept and a tangible and exciting part of student's lives.
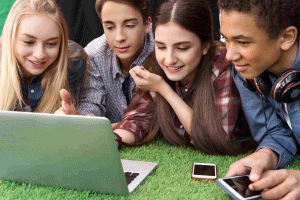
Part 3: Navigating Challenges - Addressing Variability in Data Science Teaching
Title: Navigating the Challenges: Bridging the Gap in Data Science Education
In the realm of education, the journey from well-designed standards to practical implementation in the classroom can be a complex and often challenging one. Despite our best efforts to create clear and meaningful science and math standards, particularly those incorporating data science skill development, classroom reality may diverge significantly from our intentions. The discrepancy arises from the varying levels of training and confidence that K-12 teachers have in teaching data science. Even within a single school, immense variability exists. The result is a spectrum of approaches, from enthusiastic adoption to hesitancy or avoidance of meaningful data science engagement with students.
The truth is that designing standards is just the first step. The crucial link lies in the capacity and confidence of teachers to translate these standards into impactful lessons. Unfortunately, many teachers, facing a lack of training or varying degrees of confidence, find themselves at a crossroads. Some may shy away from incorporating meaningful data science into their curriculum, fearing the unknown. Others, in their attempts, may inadvertently transfer their own misunderstandings to students, potentially doing more harm than good.
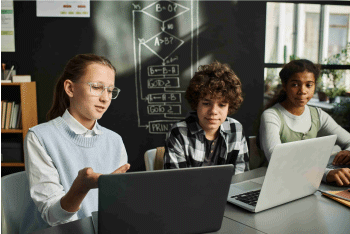
Conclusion: Paving the Way for a Data-Driven Future in Education
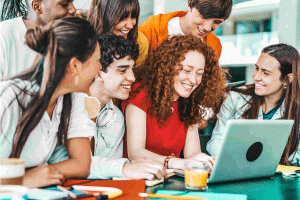
FAQs on Data Science Education in K-12:
- Why is there a need for partnerships in data science education at the K-12 level?
Partnerships between colleges, nonprofits, for-profit K- 12 ed-tech, and high schools are essential to support, implement, and sustain high-quality professional development and course development in data science. These collaborations bridge the gap and provide valuable resources for effective education in a cohesive way. - How can colleges
contribute to data science education in high schools?
Colleges can contribute by providing curriculum development support, access to experienced instructors, and exposure to real-world data science applications. These collaborations create a seamless bridge between high school and higher education. - How can nonprofits play
a role in enhancing data science education?
Nonprofits can offer funding, mentorship programs, and curriculum resources to support data science education. They act as catalysts for change, driving the integration of data science into the K-12 curriculum. How can for-profit ed-tech and industry involvement benefit data science education?
For-profit K-12 ed-tech companies can contribute to data science education by developing interactive learning platforms, offering teacher training programs, and creating adaptive technologies. Industry partnerships bring practical relevance by offering internships, guest lectures, and exposure to cutting-edge technologies. This involvement prepares students for the demands of the real world and enhances the overall learning experience.Why is teacher training crucial for successful data science education?
High-quality professional development is essential for teachers to effectively deliver data science education. Proper training ensures that students receive instruction from well-prepared and informed teachers.What role does community engagement play in data science education?
Collaboration with the local community fosters a supportive environment for data science education. Initiatives like hackathons and community projects create opportunities for students to apply their knowledge in real-life scenarios.How can educators connect abstract concepts like data science to real-world applications?
Educators can break away from traditional teaching methods by incorporating innovative approaches that make data science tangible for students. This shift sparks curiosity and enthusiasm, making learning more engaging.Why is there variability in data science teaching among K-12 teachers within a school?
The variability arises from differences in the amount of training on data science that K-12 teachers have and their confidence in teaching it. This diversity within schools can lead to varied approaches, from active adoption to avoidance of data science teaching.How can the gap in data science education for teachers be addressed?
The gap can be addressed through targeted professional development programs, resources, and mentorship opportunities. Supporting teachers with the tools and knowledge needed empowers them to integrate data science confidently.Where can educators find multivariate datasets for teaching data science in K-12?
Educators can explore platforms like Kaggle and Code.org and educational resources from organizations like NOAA and IBM Skills. These sources provide a variety of datasets suitable for K-12 students to work with and explore data science concepts.
Our Office
South Carolina, 29650,
United States